

Keep the default cluster pop-up or configure a custom pop-up to show the information you want to highlight about each cluster.For example, you can label each cluster based on two attributes, such as the average earthquake magnitude and the earthquake count, using a different label style for each attribute. To further customize cluster labels, use label classes.For example, if the layer shows parcels by their value per square foot, you can configure the cluster label to show the average value per square foot of all the points in each cluster.
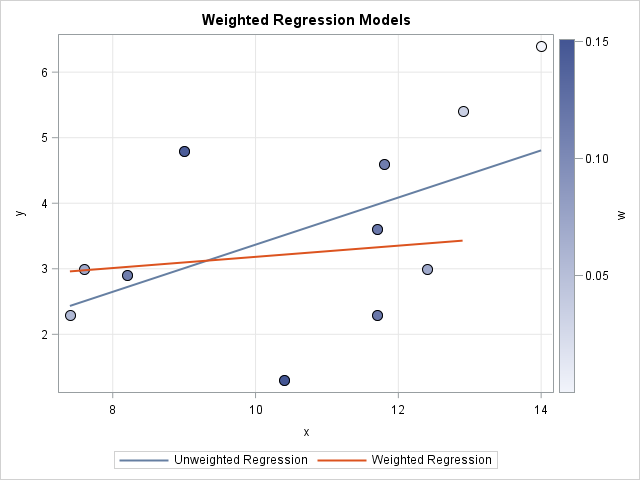
Configure labels that display the cluster count, or if the layer is styled using an attribute, use this attribute for the cluster label.You can then use the newly created summary statistics fields to style the layer or customize labels and pop-ups.
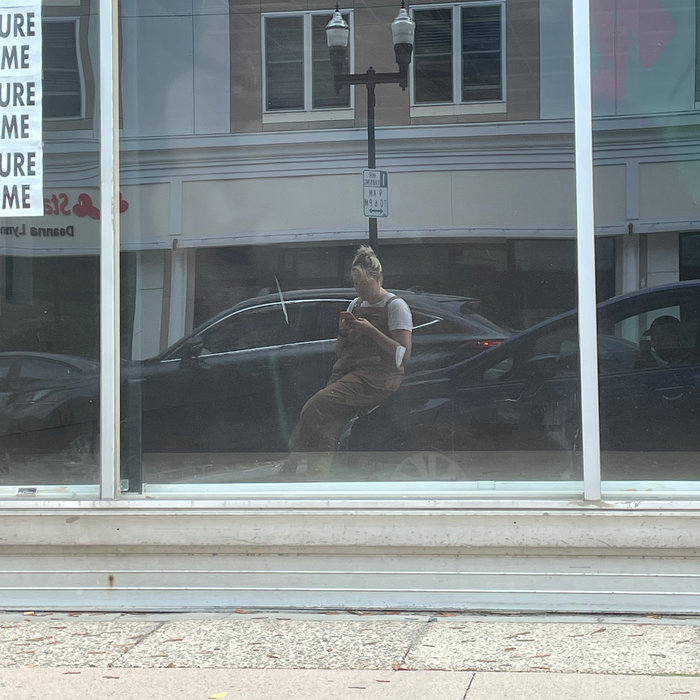
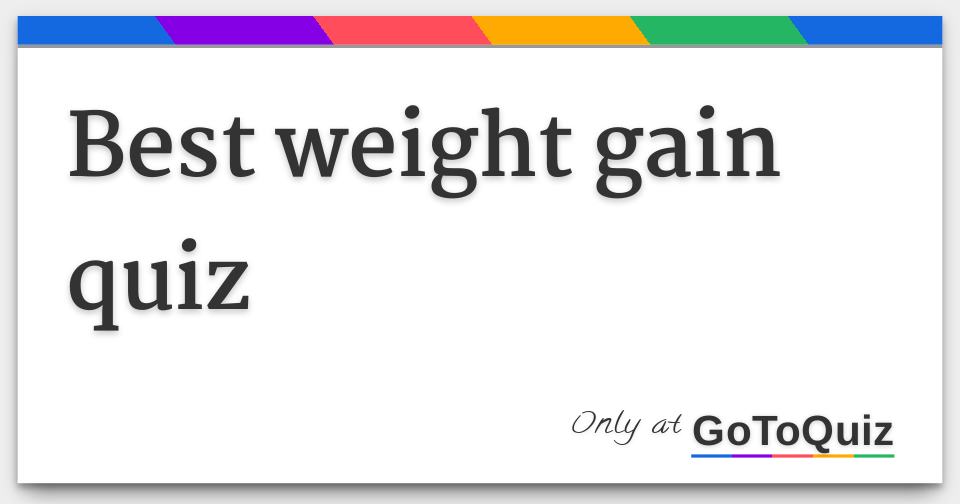
When you apply clustering to a point layer, point features that are within a certain distance of one another on the map are grouped into one symbol. It is difficult to get a realistic view of the data when many points are close together, overlap, or stack on top of each other on the map.Ĭlustering offers a quick solution to this problem. What appears to be only a few points might instead be several thousand. Layers with many point features can be deceptive. The following sections describe several methods for visualizing high-density data in Map Viewer, along with best practices for applying them. In many cases, the visual patterns revealed in these methods lead to better questions being asked of the data, which in turn require aggregation by areas for answers. Sometimes a visual effect, such as a heat map or clustering, is all that is needed. Map Viewer offers a variety of methods for extracting meaning from feature distribution in high-density datasets at multiple scales. Seeing all accidents in the country as a lot of dots on the map shows collisions do occur, but offers no insight into how, why, or where they happen. However, as you zoom out to a smaller scale, all the points merge visually, hiding the grouping you intended to show. For example, when viewing traffic collisions centered on the busiest intersection in a city, you may see multiple points grouped around the intersection. When determining the best way to represent dense data, you must first understand the scale levels at which users will typically view it. For example, a set of points may be dense at a small scale (zoomed out) but appear dispersed at a large scale (zoomed in). Use the swipe tool to compare the clustered layer to the unclustered version.įeature density is relative to the map scale. See an example of clustering for visualizing thousands of power plants in the ArcGIS Instant Apps Media app.
